The Netflix Nexus
“We are free only insofar as we exercise control over what people know about us, and in what circumstances they come to know it.”
-Timothy Snyder, On Tyranny: Twenty Lessons from the Twentieth Century
The Golden Goose:
In October 2006, Netflix announced an open competition with a grand prize of $1,000,000 in exchange for rights to a content filtering algorithm that was 10% more accurate than Netflix’ current Cinematch model. Three years later in September 2009, Team “Bellkor’s Pragmatic Chaos” used a matrix factorization model to successfully win the contest. It is this model that Netflix bases its continuously improved upon algorithm and is the foundation for the platform’s streaming recommendation services. Today, users can find thousands of personalized content recommendations within the Netflix app, a place where Netflix itself has deemed “binge-worthy”. Not only did Netflix reshape the content distribution model by elevating streaming services directly to consumers, it also revolutionized how television is given and received. Previously, television distribution occurred on a serial model, where consumers would wait weekly for new releases. Netflix, on the other hand, completely abandoned this model and instead drew inspiration from television box sets and dropped entire seasons of shows in one day— hence the idea of “binge-worthy content”. With its algorithm technology, Netflix was also able to provide users the “best options” to pick and choose from. With its greatly expansive library and its content personalization, the Netflix name has become synonymous with the idea of “infinite choice”. But this begs the question, to what extent do users of the application actually have a right to choose? When it comes to Netflix, the answer is very little. Its algorithmic technology utilizes not just individual user data in order to suggest content, but also relies on pattern recognition of the collective in order to make user aware suggestions. This allows Netflix to identify corresponding similarities between users and make better, more accurate suggestions. With the ability to make these identifications, Netflix can articulate a user selection that hides “unrelated” works. With over 5,000 titles available within the library, only a select 3,000 are shown on a user’s home page. This suggests that users miss out on roughly 2,000 available titles that Netflix has deemed irrelevant to the individual—so when choosing from suggested titles, users are making “choices” from a pool of content that has already been decided for them.
1. Money Maker
In 2020, Netflix earned roughly $24.5 billion in revenue. This is a drastic change from their 2007 revenue reports of $1.2 billion—the same year Netflix first launched streaming services. However, both these numbers reflect the company’s high retention rates, as Netflix receives all profits from streaming subscriptions alone. Being a commercial-free distributor of both third party and in-house content, Netflix relies on the subscription model especially considering their aversion to paid product placement. Proxy for paid product placement, Netflix operates on co-promotional marketing campaigns where advertisers will promote Netflix titles in exchange for embedded promotion within said title. For consumers, this means a commercial free viewing experience. For Netflix, it means total control over content production. Without the dependency of paid advertisements, Netflix is no longer bound to the constraints of an advertisers critique and can instead focus on their profit model; retention. With this in mind, it is principal to approach the Netflix sphere with a clear understanding of their intent to obtain and retain consumers for as long as possible. Netflix does not have commercial interest, they have retention interest and the algorithmic models they design and use are a direct reflection of this objective.
2. “Are You Still Watching?”
Netflix, like most tech companies, boasts a large portfolio of 962 patents to their name. These patents refer to various technologies used by the company, but this essay will explicitly focus on a select 18 that directly affect the user recommendation system. Found in the Appendix below, these 18 patents give Netflix rights to the inventions of a multitude of algorithms best described by Carlos A. Gomez-Uribe, former Vice President of Netflix Program Innovation: Personalization Algorithms, and Neil Hunt, Former Netflix Chief Product Officer. In the duo’s 2015 paper, The Netflix Recommender System: Algorithms, Business Value, and Innovation, they write, “the time that our members spend viewing Netflix content—is strongly correlated with improving retention” and thus “Our recommender system is used on most screens of the Netflix product beyond the homepage, and in total influences choice for about 80% of hours streamed at Netflix”. Their paper describes nine separate algorithms with six specifically acting as recommender programs for browsing. The Personalized Video Ranker (PVR) algorithm, for example, organizes the selection of suggested content within genre rows, so that each individual user will have their own option of content to choose from within a range of genre titles. On a broader note, the Top-N Video Rank algorithm provides the “best” content selection out of the range of the entire catalog for a user, while the Trending Now algorithm selects the most “trendy” options that also fits the user’s preferences. The Continue Watching algorithm recognizes when a user has “abandoned” certain content and will sort titles based on how likely it is the user will decidedly continue streaming it. On the other hand, Video-Video Similarity algorithms suggest content similar to that already viewed by users, within the “Because You Watched” rows of the browser. Finally, the Page Generation algorithm generates what rows will be presented to users during their browsing experience. Each of these algorithms, while somewhat similar in technique, draw upon different data sets in order to generate their suggestions allowing optimal output for consumers. An item of interest, however, are the discrepancies between Gomez-Uribe and Hunt’s work and the patents held by Netflix, specifically that of the Markov Chain. As described in Row 17 of Exhibit A, Netflix holds a patent to collect explicit and implicit information in order to determine preferred metadata tags—in short, assigned tags used to identify user interests—in order to provide better recommendations. Further, as described in Row 9 of Exhibit A, Netflix holds a patent to utilize these metadata tags in conjunction with regression models to accurately increase the likelihood of consumer interactions with media. This is done through the use of Markov chain transition probabilities. Markov chains are used to recognize the decisions a consumer will make in the future, point j, as defined by the given option of the present, point i. In other terms, the probability of a user interacting with specific content in the future is defined by what content the user is shown first, with the first content shown acting as a dependent variable. The lack of mention by Gomez-Uribe and Hunt is most likely due to the patents filing coming two years after their publication date and is likely a reflection of recent innovations of the Netflix algorithms. However, these patents are still key to note considering their explicit suggestion of Netflix’ ability to sway consumer choice while utilizing the Markov Chain. The power to accurately predict what a user needs to be shown first in order to choose the next selection, gives Netflix the opportunity to make content viewing decisions for consumers. Referring back to Netflix’ co-promotion marketing strategies for product placement, should Netflix choose to strike a deal with an advertiser to increase user viewings of certain product placement embedded content in exchange for greater promotion of title by said advertiser, what exactly is stopping them from doing so? Whether or not Netflix uses the Markov chain patent technology to do this is unclear, but it is a reality that could readily be used to their liking. It calls to question again, to what extent are users of Netflix given a right to choose? While it is arguably a consumer’s choice when making content selection, are they really given a fair opportunity? Through the use of data collection, Netflix algorithms are able to identify patterns within a user that the user themself may not be able to identify. Further, as shown by the Markov Chains, Netflix has the power to strategize the presentation of these recognized patterns in a way that identifies user’s susceptibility to decision making. Netflix is often described as the conqueror of “decision fatigue” due to their personalized recommendations, but even further, it might be because Netflix has already made the decision for you.
Disaster Capitalist
The Netflix algorithm matters because Netflix is actively pursuing an experience of network based television, ad-free. There is a clear push to emphasize Netflix Original content, even so far as a 2021 promotion of one Netflix Original film released each week of the year. Even further from this, consumers are already seeing the genesis of streaming bundles like the Disney+, Hulu and ESPN+ package. It would not be farfetched to assume that there is a possibility in the near future of consumers spending roughly $60 a month, an average estimate for the most basic cable package, for a streaming bundle that contains a multitude of platforms, “ad-free”. Figures 3.1 to 3.4 are publicly tweeted statements made by individuals either calling for, or predicting the emergence of bundled streaming.
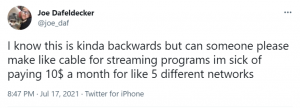
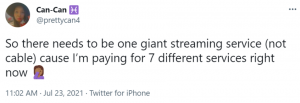
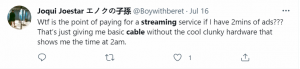
Complications with this commentary arise because streaming is not cable. Cable television, as noted in Exhibit B, offers a wide range of channels within each bundle, with the highest offering 425 channels. This is 425 separate channels, many of which belong to separate networks, with network related content. In a cable environment, users are more limited in their choices of content, when to view it and its relevance. A lack in algorithmic technology means that cable television can not personalize content for individual consumers nor predict the likelihood of user interaction, and yet Figure 3.5 suggests that the difference between streaming and cable is “cool clunky hardware that shows me the time”. This demonstrates a gap between the public’s understanding of what streaming is, the technologies it uses and the power that it holds that cable does not operate with. The “death of cable” harbors risks due to the extensive personalization technologies upheld by streaming services. When media is individualized to excessive levels, people are no longer exposed to a common world view. Users are instead provided with and associate with mindsets and ideologies that only confirm previous beliefs. Netflix and other similar streaming companies are not looking to expand worldviews, they are seeking out the algorithms that will maximize profits and expand user retention. Without regulation these companies have no motive to seek out any alternatives and the four horsemen of the streaming apocalypse—Netflix, Amazon, Disney and Warner Media—will ultimately control the narrative of all future television to come. If this means doing away with a users right to choice in exchange for higher profits, a public misperception seems like the perfect veil to hide behind.
References
Ari Levy, “The Most Liberal and Conservative Tech Companies, Ranked by Employees’ Political Donations,” CNBC, July 2, 2020, https://www.cnbc.com/2020/07/02/most-liberal-tech-companies-ranked-by-employee-donations.html
Carlos A. Gomez-Uribe and Neil Hunt. 2015. The Netflix recommender system: Algorithms, business value, and innovation. ACM Trans. Manage. Inf. Syst. 6, 4, Article 13 (December 2015), 19 pages. DOI: http://dx.doi.org/10.1145/2843948
Charlie Avery, “Netflix: The New Outlet for US Progressive Politics”, University of Birmingham, https://www.birmingham.ac.uk/research/perspective/netflix-us-progressive-politics.aspx
Mareike Jenner, Netflix and the Re-invention of Television, 5-8
Meredith Geaghan-Breiner and Kyle Desiderio, “Netflix Shows and Movies are Full of Brands—But is it Product Placement?” Insider, September 18, 2020, https://www.insider.com/product-placement-netflix-originals-shows-movies-brands-streamin-stranger-things-2020-6
Shayan Asgharnia, “Netflix’s Massive 2021 Film Slate Will Deliver New MoviesEvery Week This Year,” Variety, January 12, 2021,https://variety.com/2021/film/news/netflix-2021-new-movies-every-week-1234882900/
“2020 Annual Report”, Annual Reports and Proxies, Netflix, last modified June 3, 2021,https://ir.netflix.net/financials/annual-reports-and-proxies/default.aspx
“2007 Annual Report”, Annual Reports and Proxies, Netflix, last modified June 3, 2021,https://ir.netflix.net/financials/annual-reports-and-proxies/default.aspx
Appendix:
Exhibit B: Chart depicting cable bundle prices from top cable providers.